IntPhys 2019: A Benchmark for Visual Intuitive Physics Understanding
Riochet et al., 2020
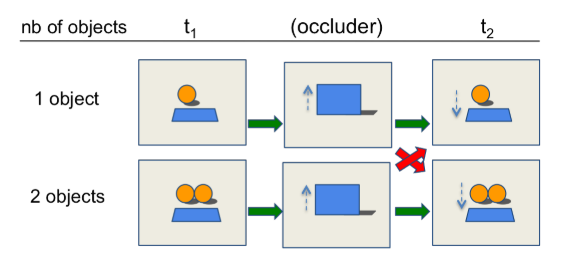
Summary
- Proposes a benchmark to evaluate how much a given system understands physics
- The system must distinguish between possible and impossible events
- Comparison of two baseline models trained with future semantic mask prediction to human perfomance demonstrates limitations of current approaches
- Links: [ website ] [ pdf ]
Background
- Artificial systems are still very limited in their ability to understand complex visual scenes
- On the other hand, infants quickly acquire an understanding of various physical concepts (e.g. object permanence, stability, gravity, etc.)
- While future prediction has been useful for training dynamics models, the prediction error is not easily interpretable
- Inspired by “violation of expectation” experiments in psychology, the IntPhys benchmark provides interpretable results by using prediciton error indirectly to choose between possible and impossible events
- This also has the benefit of enabling rigorious human-machine comparisons
- The system required to output a plausibility score for each video
Methods
- Tests for three basic physical concepts: object permanence (O1), shape constancy (O2), and spatio-temporal continuity (O3)
- Design principles:
- Well matched sets to minimize low-level biases
- Parametric stimulus complexity: visible/occluded, object motion, number of objects
- Procedurally generated variablity: object shapes, textures, distances, trajectories, occluder motion, camera position
- Metrics:
- Relative error: within a set, possible movies are more plausible
- Absolute error: globally, possible movies are more plausible
- Dataset:
- Train: 15K videos of possible events (~7 seconds each)
- Test: three blocks with 18 scenarios, 200 renderings of each scenario, objects and textures are present in training set
- Additional depth, object segmentation, 3D position (train only), camera position (train only), and object linking info (train only) available
Results
- Baselines:
- CNN encoder-decoder
- GAN
- Trained to predict future semantic mask at two different time horizons: 5 frames and 35 frames
- Preliminary models trained with predictions at the pixel level failed to produce convicing object motions
- Models perform poorly when impossible events are occluded, even the long-term prediciton models
- Humans significantly outperform models across scenarios
- Except for O3 (spatio-temporal continuity) occluded, where humans also perform near chance
Conclusion
- IntPhys provides a well-designed benchmark for evaluting a system’s understanding of a few core concepts about the physics of objects
- The relative success of semantic mask prediction versus pixel prediction suggests a benefit in operating at a more abtract level