Intuitive experimentation in the physical world
Bramley et al., 2018
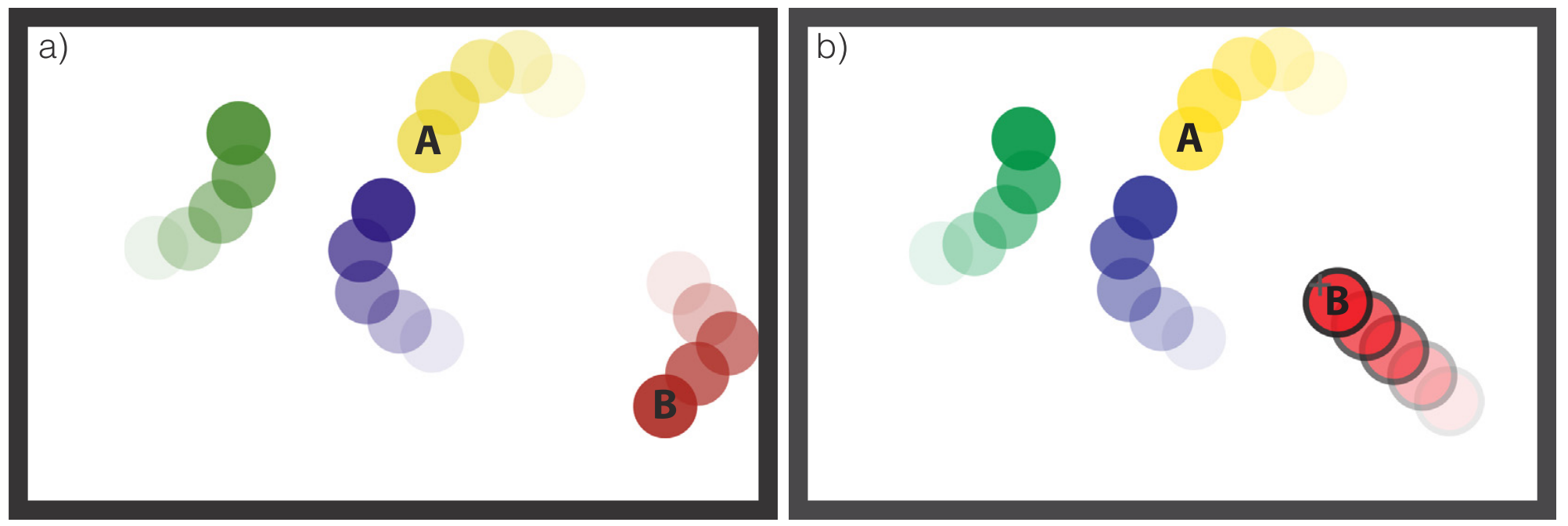
Summary
- How do people actively experiment to discover latent properties within the physical world?
- Performed experiments where participants interacted with objects in order to identify the masses or attractive/repulsive forces that governed their movement
- The interactions selectively produced evidence that revealed the physical property being investigated
- Active learners produced more accurate inferences than passive and yoked observers
- Links: [ website ]
Background
- From a causal perspective, actions can be seen as interventions which help reveal how to world works
- Unclear how these actions are chosen, and how complex and informative they are
- Active learning studies in humans usually focus on differentiating between a relatively small number of discrete hypotheses
- Evidence for its utility has also been mixed
- This study explores active learning in a continuously dynamic environment
- Learner must decide where, how, and when to intervene
- Prior work using a similar simulated environment demonstrated that humans are worse at detecting attraction versus repulsion when passively observing
- Divergence corresponds to asymmetry in evidence, since objects that repel each other rarely spend enough time close together
Methods
- Aim to establish how effective participants’ actions are at reducing uncertainty about the properties they’re asked about
- 2D microworld environment - contains a number of colored pucks with varying masses and local (magnet-like) pair-wise forces
- Active: allow participants to grab objects by clicking on them and dragging
- Passive: participants only observe the world
- Yoked: passively observe actions of active participant
- Ideal Observer (IO): predicts how the scene would unfold given various assumptions about the properties and comparing to whats observed
- Predictive Divergence (PD): take divergence between simulations varying the property and what is observed, averaging over all possible settings for other properties
Results
- Just looking at accuracy, active participants were significantly better than passive and yoked participants, which performed similarly
- Active participants’ performance was moderately predictive of their yoked counterparts’ (r=0.49)
- In general, participants were worse at inferring masses compared to forces
- Active learners benefited the most for inferring repulsion
- Yoked participants had marginal improvement in inferring forces
- While active participants generated the same amount of evidence overall as passive participants, they generated more evidence about force
- PD for force and mass of target was higher for active participants compared to passive, also for intervening periods of active participants compared to observing periods
- Giving the same learning goal to yoked participants raises their performance to the level of their active counterparts
Conclusion
- Provides additional evidence for the importance of active learning and volitional control of interventions
- How these sophisticated strategies are learned remains an open question