Could a Neuroscientist Understand a Microprocessor?
Jonas and Kording, 2017
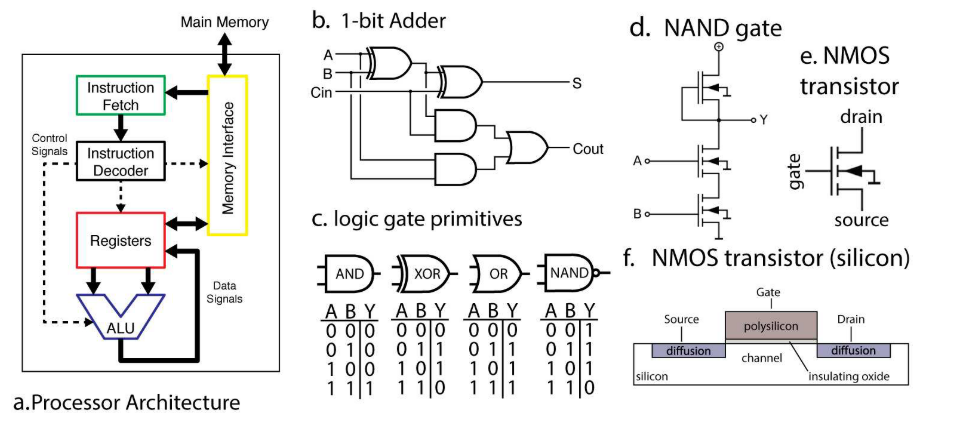
Summary
- Addresses a popular belief in neuroscience that the field is primarily data limited, and producing large, multimodal datasets will lead to fundamental insights into the brain
- Use a classical microprocessor as a model organism and apply modern data analysis methods from neuroscience to understand its information processing
- These approaches reveal structure in the data but do not meaningfully explain information processing in the microprocessor
- Argue that neuroscientists should use complex non-linear dynamical systems with known ground truth (e.g. a microprocessor) to validate their data analysis methods
- Links: [ website ]
Background
- Difficult to evaluate effectiveness of various analysis methods in neuroscience due to lack of ground truth
- A known artificial system, the microprocessor, can act as a testbed for neuroscience methods
- Inspired by Yuri Lazbnick’s critique of modeling in molecular biology, “Could a biologist fix a radio?”
Methods
- Use MOS 6502 as “model organism”, with three “behaviors” (games): Donkey Kong, Space Invaders, and Pitfall
- Analogous to connectomics work, identify circuit structures and produce a transitor-accurate netlist, supporting a cycle-accurate simulator
- 3510 transistors total
- What does an understanding look like? Hierarchical description of the functional modules
- ALU, byte wide adder, binary adders, logic gates, transistors for the processor
- Regions, circuits, microcircuits, neurons, synapses in the brain
Results
- Applying connectomics techniques reveal various transistor “types”, but in reality only one physical “type” of transistor
- No way to go from anatomy to function, besides basic cell-type clustering
- Lesioning single transistors at a time demonstrates that a subset of the transistors are necessary for running the game (“behavior”)
- Does this mean they’ve found “Donkey Kong transistors”?
- Plotting the “spike rate” of transistors as a function of the brightness of the most recently displayed pixel produces a few transistors with a strong tuning
- Transistors relate in highly non-linear ways to the brightness of the screen
- Other methods like analyzing correlations, local field potentials, Granger causality, and dimensionality reduction likewise don’t produce satisfactory explanations
Conclusion
- Doesn’t actually present any potentially better methods
- Arguement for using systems with known ground truth to validate methods seems reasonable
- The “behavior” used is entirely passive